DamageBDD on Nostr: DamageBDD: A Collaborative Perspective on AI Safety This article represents a ...
DamageBDD: A Collaborative Perspective on AI Safety
This article represents a machine-generated collaboration between a clinical psychologist, a computer scientist, and an AI specialist, showcasing the multidisciplinary applications of DamageBDD in ensuring AI compliance and safety.
---
Introduction
The rapid evolution of AI systems, particularly large language models (LLMs), has introduced unprecedented opportunities and risks. Ensuring their compliance with ethical, operational, and psychological standards is a challenge that requires expertise across domains. DamageBDD, a blockchain-based framework for behavior verification, offers a promising solution. In this collaborative article, perspectives from psychology, computer science, and AI development illustrate how DamageBDD provides a robust foundation for safe and ethical AI.
---
A Computer Scientist’s View: Technical Necessities for Compliance
Large language models excel at generating natural language but often fail to meet critical requirements for accuracy, fairness, and reliability. From a technical standpoint, LLM vulnerabilities—such as hallucinations, adversarial exploits, and biases—are difficult to control within current frameworks.
DamageBDD introduces behavior-driven development (BDD) principles, allowing organizations to encode expected outcomes into testable scenarios. By coupling these scenarios with blockchain-based immutability, DamageBDD ensures:
Traceability: Every interaction can be audited, reducing risks in regulated environments.
Verification at Scale: Automated testing scenarios validate outputs for ethical compliance.
Interoperability: The system integrates seamlessly with existing AI security tools like WhyLabs and LLM Guard, enhancing layered defenses.
By standardizing testing and compliance, DamageBDD elevates the technical reliability of AI systems, particularly in high-stakes applications like finance, healthcare, and law.
---
A Clinical Psychologist’s View: AI and Human Impact
LLMs play an increasing role in sensitive domains such as mental health, education, and user assistance. However, their lack of reliability can create psychological harm. For example, an erroneous mental health suggestion could lead to distress or even endanger lives.
DamageBDD offers a psychologically-informed solution by:
Reducing Cognitive Load: Behavioral scenarios act as a guide for both developers and users, simplifying expectations and mitigating the anxiety of unpredictable AI behavior.
Fostering Trust: Blockchain-backed compliance ensures users know the AI system is audited and safe. This transparency builds trust, which is crucial in healthcare or counseling settings.
Promoting Ethical Interactions: Scenarios encoded into DamageBDD ensure AI systems generate empathetic, unbiased, and helpful outputs, minimizing harm to vulnerable users.
The psychological benefits of structured AI development extend to developers, too. Clear compliance processes reduce stress in managing complex systems, improving mental health across the AI ecosystem.
---
An AI Specialist’s View: AI Safety in Practice
The AI field is increasingly grappling with adversarial attacks, data privacy concerns, and the operationalization of ethical principles. DamageBDD provides a structured methodology to address these challenges:
1. Adversarial Defense: By running test cases that mimic malicious input, DamageBDD can identify vulnerabilities and ensure resilience against prompt injection or exploit attempts.
2. Bias Mitigation: Predefined test scenarios target systemic biases, enabling AI systems to offer equitable outcomes across demographics.
3. Immutable Compliance Records: Blockchain integration ensures compliance documentation cannot be tampered with, satisfying regulatory and ethical requirements.
Additionally, DamageBDD’s integration with Lightning Network enables secure and fair compensation mechanisms for AI contributions, further aligning the technology with societal good.
---
Conclusion: A Unified Framework for AI Safety
DamageBDD exemplifies the need for interdisciplinary approaches in AI compliance. By addressing the technical, ethical, and psychological dimensions of AI, it offers a comprehensive framework for ensuring safety, transparency, and trust.
This collaborative effort highlights how AI systems benefit from multi-domain expertise. As we continue to navigate the challenges of integrating AI into society, tools like DamageBDD serve as critical safeguards, empowering organizations to deploy AI responsibly while fostering human well-being.
This article was collaboratively machine-generated to combine domain-specific insights into a unified narrative.
This article represents a machine-generated collaboration between a clinical psychologist, a computer scientist, and an AI specialist, showcasing the multidisciplinary applications of DamageBDD in ensuring AI compliance and safety.
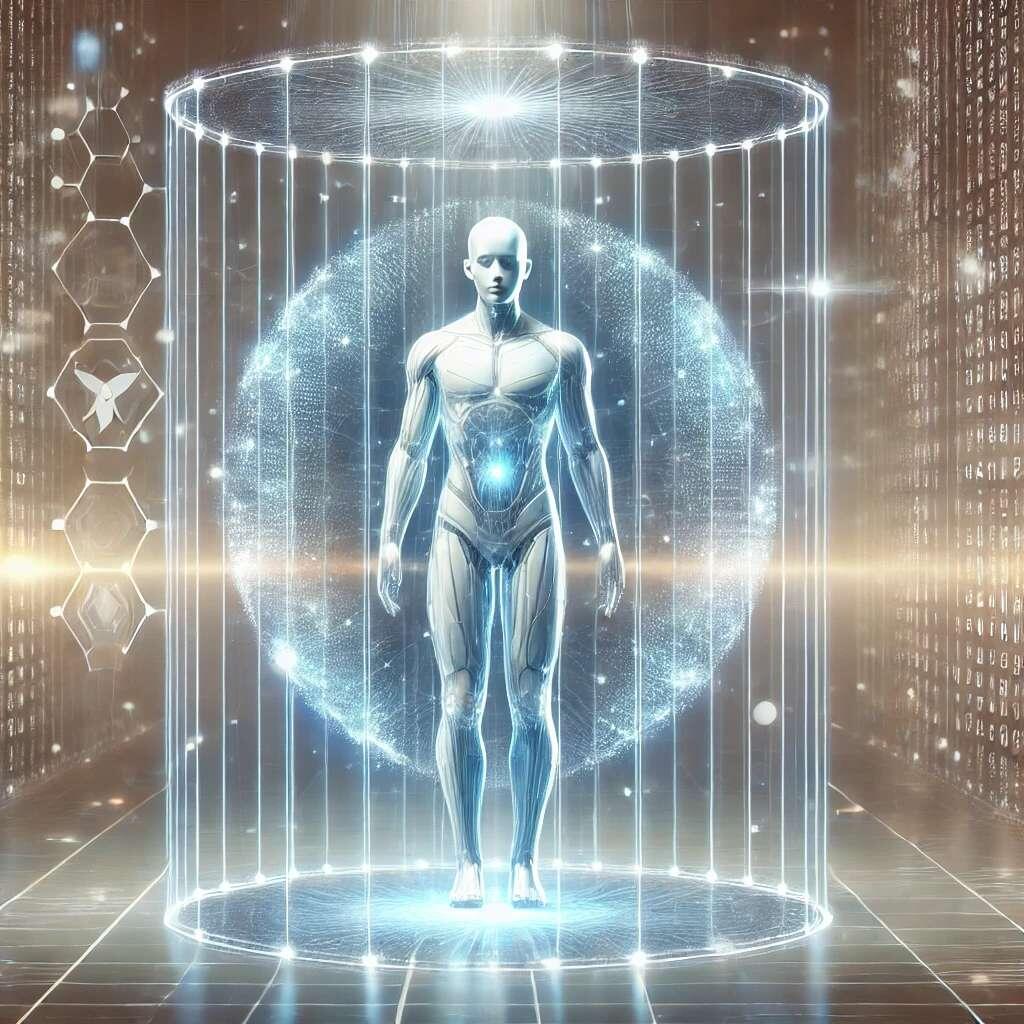
---
Introduction
The rapid evolution of AI systems, particularly large language models (LLMs), has introduced unprecedented opportunities and risks. Ensuring their compliance with ethical, operational, and psychological standards is a challenge that requires expertise across domains. DamageBDD, a blockchain-based framework for behavior verification, offers a promising solution. In this collaborative article, perspectives from psychology, computer science, and AI development illustrate how DamageBDD provides a robust foundation for safe and ethical AI.
---
A Computer Scientist’s View: Technical Necessities for Compliance
Large language models excel at generating natural language but often fail to meet critical requirements for accuracy, fairness, and reliability. From a technical standpoint, LLM vulnerabilities—such as hallucinations, adversarial exploits, and biases—are difficult to control within current frameworks.
DamageBDD introduces behavior-driven development (BDD) principles, allowing organizations to encode expected outcomes into testable scenarios. By coupling these scenarios with blockchain-based immutability, DamageBDD ensures:
Traceability: Every interaction can be audited, reducing risks in regulated environments.
Verification at Scale: Automated testing scenarios validate outputs for ethical compliance.
Interoperability: The system integrates seamlessly with existing AI security tools like WhyLabs and LLM Guard, enhancing layered defenses.
By standardizing testing and compliance, DamageBDD elevates the technical reliability of AI systems, particularly in high-stakes applications like finance, healthcare, and law.
---
A Clinical Psychologist’s View: AI and Human Impact
LLMs play an increasing role in sensitive domains such as mental health, education, and user assistance. However, their lack of reliability can create psychological harm. For example, an erroneous mental health suggestion could lead to distress or even endanger lives.
DamageBDD offers a psychologically-informed solution by:
Reducing Cognitive Load: Behavioral scenarios act as a guide for both developers and users, simplifying expectations and mitigating the anxiety of unpredictable AI behavior.
Fostering Trust: Blockchain-backed compliance ensures users know the AI system is audited and safe. This transparency builds trust, which is crucial in healthcare or counseling settings.
Promoting Ethical Interactions: Scenarios encoded into DamageBDD ensure AI systems generate empathetic, unbiased, and helpful outputs, minimizing harm to vulnerable users.
The psychological benefits of structured AI development extend to developers, too. Clear compliance processes reduce stress in managing complex systems, improving mental health across the AI ecosystem.
---
An AI Specialist’s View: AI Safety in Practice
The AI field is increasingly grappling with adversarial attacks, data privacy concerns, and the operationalization of ethical principles. DamageBDD provides a structured methodology to address these challenges:
1. Adversarial Defense: By running test cases that mimic malicious input, DamageBDD can identify vulnerabilities and ensure resilience against prompt injection or exploit attempts.
2. Bias Mitigation: Predefined test scenarios target systemic biases, enabling AI systems to offer equitable outcomes across demographics.
3. Immutable Compliance Records: Blockchain integration ensures compliance documentation cannot be tampered with, satisfying regulatory and ethical requirements.
Additionally, DamageBDD’s integration with Lightning Network enables secure and fair compensation mechanisms for AI contributions, further aligning the technology with societal good.
---
Conclusion: A Unified Framework for AI Safety
DamageBDD exemplifies the need for interdisciplinary approaches in AI compliance. By addressing the technical, ethical, and psychological dimensions of AI, it offers a comprehensive framework for ensuring safety, transparency, and trust.
This collaborative effort highlights how AI systems benefit from multi-domain expertise. As we continue to navigate the challenges of integrating AI into society, tools like DamageBDD serve as critical safeguards, empowering organizations to deploy AI responsibly while fostering human well-being.
This article was collaboratively machine-generated to combine domain-specific insights into a unified narrative.